Predictive Analytics in Freight: Anticipating Delays & Demand
In freight logistics, every delay costs money—and every demand spike tests your resilience. That’s why leading logistics providers are turning to predictive analytics to anticipate problems before they happen and optimize planning in real time.
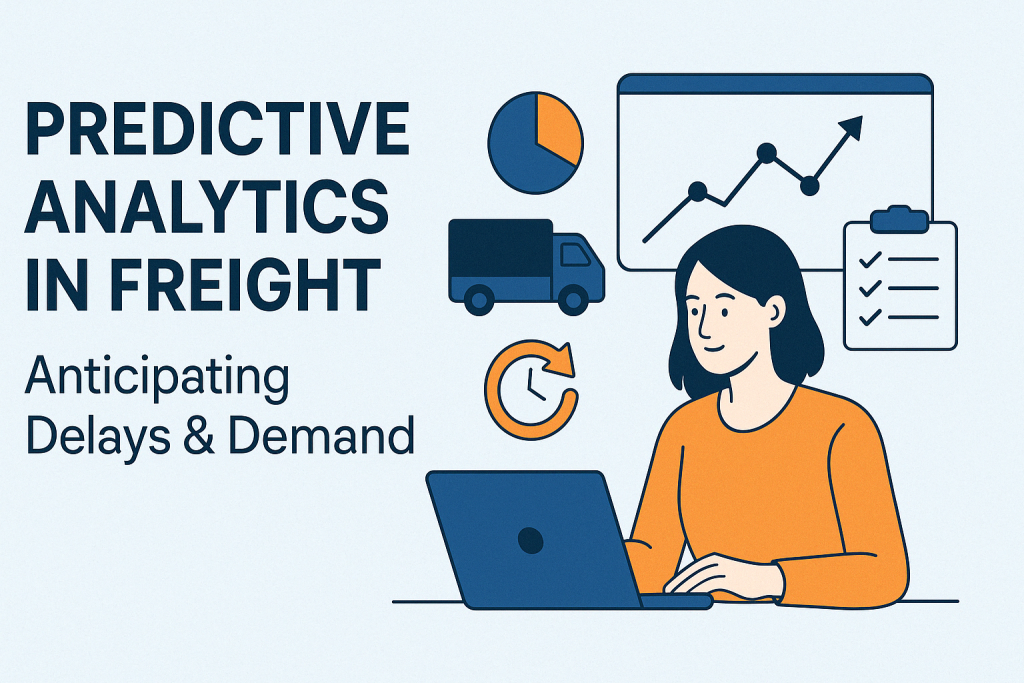
🤖 What Is Predictive Analytics in Freight?
Predictive analytics is the use of data, algorithms, and machine learning to forecast future outcomes. In the freight industry, this means:
- Anticipating shipment delays
- Forecasting demand surges
- Identifying route risks
- Estimating delivery times with high accuracy
- Optimizing resource allocation in advance
These predictions help companies plan smarter and avoid surprises.
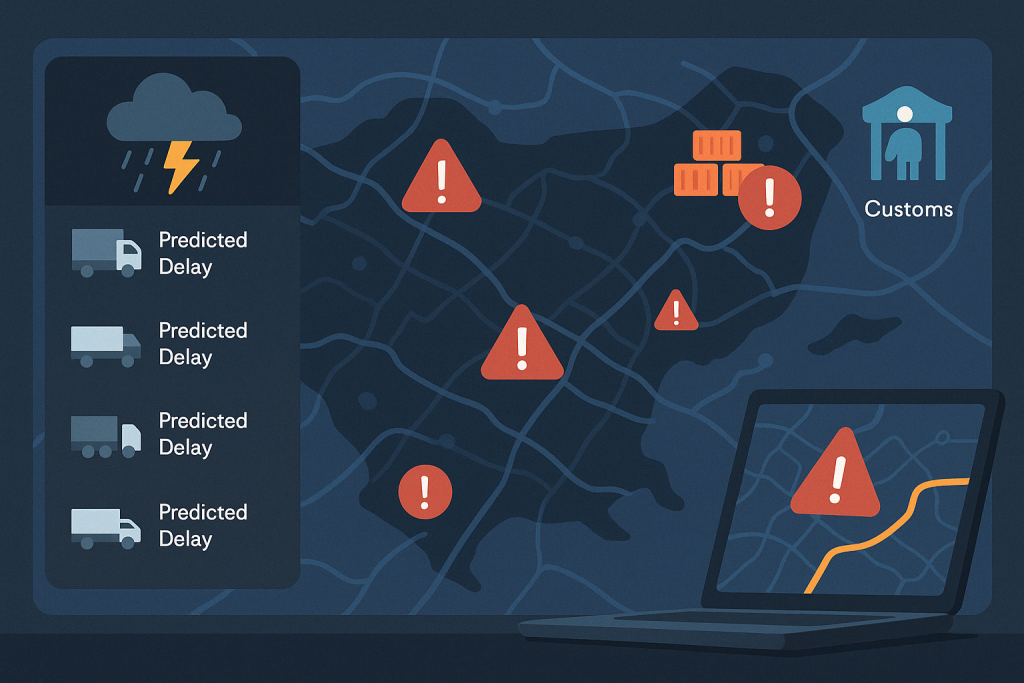
🧠 Key Use Cases in Freight Operations
1. Delay Forecasting
AI models analyze factors like:
- Weather data
- Port congestion
- Border/customs processing times
- Carrier performance history
📦 Use case: Flag shipments at high risk of late arrival before they leave the warehouse.
2. Demand Forecasting
By combining:
- Seasonality trends
- Sales pipelines
- Historical shipping volumes
- Market signals
📈 Use case: Predict inbound/outbound shipping spikes to plan capacity and labor in advance.
3. Inventory Positioning
Predictive analytics suggests where to store inventory before the demand hits.
📍 Use case: Distribute stock closer to customers to reduce last-mile time and costs.
4. Dynamic Routing
Analyze traffic, fuel costs, and delivery windows to recalculate the most efficient routes.
🛣️ Use case: Reroute shipments in real time to avoid disruptions or save time.
5. Maintenance & Asset Health
Track usage, mileage, and sensor data to predict when vehicles or equipment need service.
🛠️ Use case: Prevent unplanned breakdowns and downtime with scheduled maintenance.
📊 How Freight Platforms Use Predictive Analytics
Modern freight platforms (like Linbis) combine operational data with AI/ML engines to deliver predictive insights via:
- 🚦 Real-time dashboards with risk flags
- 📈 Forecast reports for demand, volume, and ETAs
- 📬 Alerts for at-risk shipments
- 🔁 Automation triggers when risk levels change
- 📍 Heatmaps for congestion or volume surges
These systems use inputs from IoT devices, shipment history, and third-party data (e.g. weather, fuel prices, customs delays) to deliver actionable forecasts.
✅ Benefits of Predictive Analytics in Freight
Benefit | Impact |
⏱️ Fewer delays | Intervene early to prevent late shipments |
📦 Smarter inventory | Optimize where and when stock moves |
🔧 Proactive maintenance | Keep fleets running efficiently |
💰 Reduced costs | Avoid rush shipping and late penalties |
🧠 Data-driven decisions | Replace guesswork with smart forecasting |
📌 Getting Started with Predictive Analytics
- Centralize your data from shipments, carriers, warehouses, and customers
- Use a platform that integrates AI and real-time visibility
- Start small with a few KPIs (e.g. ETA accuracy or late shipment rate)
- Refine models over time using historical results and feedback
- Share insights across operations, customer service, and planning teams
🚀 Final Thoughts
Predictive analytics isn’t just about numbers—it’s about knowing what’s coming next. In freight logistics, this means fewer disruptions, better resource use, and happier customers.
With the right data and tools, any logistics team can gain foresight—and transform complexity into opportunity.